2024 Highlights from Biostatistics and Health Data Science Research
In the fields of biostatistics and health data science, our faculty members are constantly pushing the boundaries of knowledge and application. This section proudly presents a curated collage of their groundbreaking work published in 2024. Each entry provides a glimpse into the innovative research undertaken by our faculty, and their potential impact in understanding and solving complex health-related challenges. Let's celebrate their achievements and the contributions to medicine, public health, policy-making, and beyond.
Qixuan Chen, PhD
Measurement error is a common challenge in environmental epidemiologic studies, but methods for addressing this issue in regression models with multiple environmental exposures as covariates have not been well investigated. To address this gap, Dr. Qixuan Chen, working with her student Yuanzhi Yu and collaborators, developed a constrained multiple imputation (MI) approach, which outperforms existing methods, producing estimated regression coefficients with reduced bias and confidence intervals that have close to the nominal level coverage. The MI method offers two advantages over the alternative methods for handling measurement error. First, it enables the imputation of not only error-free exposure measures but also nondetects in the error-prone exposures, as well as any missing values in other error-free covariates. Second, the MI approach is highly adaptable and can be extended to more complicated models through modifications to the imputation models. The utility of this method was demonstrated in estimating the association between co-exposure to multiple indoor allergens and asthma morbidity among asthmatic children in New York City.

Yu, Y., Little, R. J., Perzanowski, M., & Chen, Q. (2024). Multiple imputation of more than one environmental exposure with nondifferential measurement error. Biostatistics (Oxford, England), 25(2), 306–322. https://doi.org/10.1093/biostatistics/kxad011
Jeff Goldsmith, PhD
Accelerometers and other wearable devices can provide round-the-clock monitoring of a broad range of physical activity behaviors. Historically, these rich observations were distilled to a few simple summaries like total activity. More recently, researchers have developed ways to capture average daily patterns; however, these are unable to quantify activity in low- or high-intensity ranges. In work lead by Alvaro Mendez-Civieta, Jeff Goldsmith developed quantile-based approaches that identify person-specific daily activity patterns across a range of intensities.
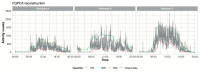
Álvaro Méndez-Civieta, Ying Wei, Keith M. Diaz, Jeff Goldsmith, Functional quantile principal component analysis, Biostatistics, 2024;, kxae040, https://doi.org/10.1093/biostatistics/kxae040
Prakash Gorroochurn, PhD
Dr. Gorroochurn has spent the last 6 years researching the development of evolutionary genetics. This has resulted in his writing of a comprehensive book on the subject entitled “The Development of Evolutionary Genetics: From Early Ideas on Evolution to the Modern Synthesis”. The book will be published by Springer in early 2025 and covers more than 800 pages of detailed historical material. Dr Gorroochurn’s future research will involve population structure in population genetics and the use of exchangeability in statistics.
Tian Gu, PhD
In 2024, Dr. Tian Gu focused on developing novel statistical and machine learning methods in transfer learning to address challenges in combining high-dimensional data from heterogeneous sources. This work spanned both theoretical advancements in top statistical journals and practical applications to electronic health record data. Dr. Gu was also recognized for excellence in Biometrics Peer Reviewing at the 2024 International Biometric Conference.
Wenpin Hou, PhD
Cell type annotation is a fundamental step in single-cell RNA sequencing (scRNA-seq) analysis. This process is often laborious and time-consuming, requiring a human expert to compare genes highly expressed in each cell cluster with canonical cell type marker genes. Although automated cell type annotation methods have been developed , manual annotation using marker genes remains widely used. Houand Ji demonstrated that the large language model GPT-4 can accurately annotate cell types using marker gene information in single-cell RNA sequencing analysis. When evaluated across hundreds of tissue and cell types, GPT-4 generates cell type annotations exhibiting strong concordance with manual annotations. This capability can considerably reduce the effort and expertise required for cell type annotation. Additionally, they have developed an R software package GPTCelltype for GPT-4’s automated cell type annotation.
This work has been featured in Columbia News Spotlight, Columbia MSPH News, Science Daily, The Medical News, Health Tech World, and 6 other news outlets. It ranked the top #1 when comparing to 69 others from the same source and published within six weeks. This work has been reviewed in Nature Methods Embedding AI in biology and Toward learning a foundational representation of cells and genes. As of May 2024 , this highly cited paper received enough citations to place it in the top 1% of the academic field of Biology & Biochemistry based on a highly cited threshold for the field and publication year. With the Altmetric Attention Score 284, it ranked the top #1 when comparing to 75 others from the same source and published within six weeks.

Hou, W., & Ji, Z. (2024). Assessing GPT-4 for cell type annotation in single-cell RNA-seq analysis. Nature methods, 21(8), 1462–1465. https://doi.org/10.1038/s41592-024-02235-4Software package: GPTCelltype.
Zhonghua Liu, ScD
Dr. Liu's research below will appear in Cell Genomics in December 2024.

Highlights:
- MR-SPI is a new instrumental variable method for causal inference in proteomics data
- Seven plasma proteins have been identified as linked to Alzheimer’s disease
- AlphaFold3 predicts protein 3D structural changes caused by missense mutations
- Genetic variant-induced structural changes may help identify future drug targets
eTOC Blurb:
Yao et al. (2024) develop a computational pipeline integrating a robust Mendelian randomization method with AlphaFold3 to select valid pQTL instruments, identify causal protein biomarkers, and predict 3D structural alterations. Seven plasma proteins have been identified as linked to Alzheimer’s disease and may aid future drug development.
Daniel Malinsky, PhD
Daniel Malinsky has been studying the data-driven selection of causal graphical models using constraint-based algorithms, which determine the existence or non-existence of edges (causal connections) in a graph based on testing a series of conditional independence hypotheses. Sometimes the role of graphical model selection is to narrow down a graph that will inform the estimation of some specific causal effect, for example, in an observational epidemiological study that aims to quantify the effect of an exposure on an outcome but with substantial uncertainty about what are the right set of covariates to “adjust” for. In this context, Dr. Malinsky argues that a “cautious” approach to graph selection should control the probability of falsely removing edges and prefer dense, rather than sparse, graphs. In a new paper, he proposes a simple inversion of the usual conditional independence testing procedure: to remove an edge, test the null hypothesis of conditional association greater than some user-specified threshold (an equivalence test), rather than the null of independence. This equivalence testing formulation to testing independence constraints leads to a procedure with that selects dense graphs and has desirable statistical properties that better match the inferential goals of observational epidemiological studies.

D. Malinsky (2024) “A cautious approach to constraint-based causal model selection." Submitted. arXiv:2404.18232
Christine Mauro, PhD & Melanie Wall, PhD
Medications for opioid use disorder (MOUD) are considered the first line treatment for opioid use disorder. As states expanded Medicaid beginning in 2014 under the Affordable Care Act, policymakers and public health officials have been interested in the potential for expansion to increase access to MOUD. With collaborators, Dr. Mauro and Dr. Wall used data from the Treatment Episode Data Set – Admissions to examine whether there were changes in MOUD use in Medicaid expansion states compared to non-expansion states. Difference-in-Difference models that accounted for the varying years of state expansion and potential heterogenous treatment effects across time were used to estimate the effect of expansion on MOUD use. Results showed there was a 6.4 percentage point (95 % CI: −0.01–13.0) increase in the probability of receiving MOUD among individuals receiving care after expansion (compared to the pre-expansion period). They also discovered that there was tremendous variability among states in the change in probability of receiving MOUD from prior to after Medicaid expansion from an almost 30 percentage point increase in New York to an almost 20 percentage point decrease in Washington, DC.

Presskreischer, R., Mojtabai, R., Mauro, C., Zhang, Z., Wall, M., & Olfson, M. (2024). Medicaid expansion and medications to treat opioid use disorder in outpatient specialty care from 2010 to 2020. Journal of substance use and addiction treatment, 168, 209568. Advance online publication. https://doi.org/10.1016/j.josat.2024.209568
Ian McKeague, PhD
Dr. Ian McKeague and collaborators have been developing a nonparametric inference framework for occupation time curves derived from wearable device data. Such curves provide the total time a subject maintains activity above a given level as a function of that level. Taking advantage of the monotonicity properties of these curves, we develop a likelihood ratio approach to constructing confidence bands that give simultaneous coverage over a range of activity levels. Application to wearable device data from an ongoing study of an experimental gene therapy for mitochondrial DNA depletion syndrome is also a focus of this research.

Caleb Miles, PhD
Trials for the treatment of psychiatric and substance use disorders can be difficult, time-consuming, and expensive to conduct, and partially as a consequence, have sample sizes that may be underpowered for: 1) detecting moderately sized average treatment effects (ATEs) that may nonetheless be important for health at the population level, and 2) learning optimal individualized treatment rules (i.e., rules that match treatments to individuals based on demographic and clinical characteristics that optimize outcomes of interest), which are the cornerstone of personalized medicine. Data fusion is a relatively new and increasingly popular domain of data science that combines data from multiple studies to improve statistical power and answer questions that cannot be addressed by a single study alone. Dr. Miles is developing methods to harness follow-up and other post-exposure measurements as potential proxies for outcomes that are systematically missing by study to deliver more precise estimates of causal treatment effects and facilitate the learning of treatment rules that maximize benefits and reduce harms.
Todd Ogden, PhD
Dr. Todd Ogden has been working for many years on the analysis of complex data objects, with particular interest in functional data, i.e., data that can be regarded as being a function of some other variable. Interested in the problem of building prediction models based on functional data objects, Dr. Ogden and a collaborator turned to the well-known support vector machine (SVM). The SVM is a robust prediction model, but it cannot take into account high correlations between repeated measurements, nor can it be used for irregular data. They proposed a novel method to integrate functional principal component analysis with SVM techniques for classification and regression to account both for the continuous nature of functional data and for any nonlinear relationship between the scalar response variable and the functional predictors. They showed that these methods have considerable advantages over alternative approaches, especially when the measurement errors in the functional predictors are relatively large.

Xie, S., & Ogden, R. T. (2024). Functional support vector machine. Biostatistics (Oxford, England), 25(4), 1178–1194. https://doi.org/10.1093/biostatistics/kxae007
Martina Pavlicova, PhD
Martina Pavlicova developed and implemented the "Integrative Capstone Experience," a unique course designed to bridge academic learning with practical application in biostatistics. Now in its second successful year, the course challenges MPH students to analyze real-world data using multiple advanced analytical methods, critically comparing their results to gain deeper insights. This comparative approach fosters advanced problem-solving skills, encouraging students to think beyond standard applications and equipping them to tackle complex challenges in public health and medicine.
Linda Valeri, PhD
In survival settings, competing events refer to any event that makes it impossible for the event of interest to occur. Not accounting for competing events can lead to substantial biases caused by the fact that individuals might die and do not have the opportunity to experience the event of interest. For example, in health disparities research there is interest in evaluating whether disparities in cancer survival might be due to delays in receiving treatment. However, individuals might die before being treated, which introduces the competing risk issue. Dr. Valeri has introduced a new approach for causal mediation analysis with time-to-event mediators and outcomes in the presence of competing risks, and has recently released the associated software for ease of implementation. One of the key differences between this approach and previously considered approaches to deal with competing events is that it does not require the elimination of the competing event, nor it requires the exposure to be separated in components that only affect the terminal event, as these scenarios are not realistic in heath disparities research. On the other hand, it provides new definitions of direct and indirect effects that accommodate the effect of the exposure on the competing event.

Valeri, L., Proust-Lima, C., Fan, W., Chen, J. T., & Jacqmin-Gadda, H. (2023). A multistate approach for the study of interventions on an intermediate time-to-event in health disparities research. Statistical methods in medical research, 32(8), 1445–1460. https://doi.org/10.1177/09622802231163331
Wang, Z., Shi, B., Proust-Lima, C., Jacqmin-Gadda, H., & Valeri, L. (2025). Multistate approach for stochastic interventions on a time-to-event mediator in the presence of competing risks: A new R command within the CMAverse R package. Epidemiology (Cambridge, Mass.), 36(1), 139–140. https://doi.org/10.1097/EDE.0000000000001791
Shikun Wang, PhD
Dr. Shikun Wang and her collaborators developed a novel longitudinal varying coefficient single-index model for analyzing cancer-related medical costs over time using patient characteristics. This model will be strategically useful for health policy researchers to understand cost trajectories and their drivers, enabling tailored policy decisions and personalized healthcare strategies. It also facilitates the analysis of censored survival data, with broad prospects for applications in medical cost research and health economics.
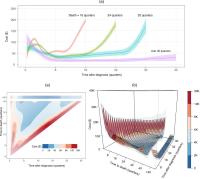
Wang, S., Ning, J., Xu, Y., Shih, Y. T., Shen, Y., & Li, L. (2024). Longitudinal varying coefficient single-index model with censored covariates. Biometrics, 80(1), ujad006. https://doi.org/10.1093/biomtc/ujad006
Shuang Wang, PhD
We recently developed a comprehensive clinical diagnosis tool, GCN-EPI, which is a Graph Convolutional Network using EHR and genetic data with Patient graph Integration. GCN-EPI integrates both EHR and omics data while accounting for the heterogeneity between the two modalities. GCN-EPI learns patient representations based on patients’ EHR data and uses neighbor information from a patient graph being generated using both high-dimensional omics data and EHR data. GCN-EPI uses a one-step fashion and learns patient representations to optimize a prediction loss, thereby enhancing the predictive capabilities of clinical diagnosis tools.
Yuanjia Wang, PhD
Reinforcement learning models for behavioral tasks
Major depressive disorder (MDD) is one of the leading causes of disability-adjusted life years. Emerging evidence indicates the presence of reward processing abnormalities in MDD. An important scientific question is whether the abnormalities are due to reduced sensitivity to received rewards or reduced learning ability. Motivated by the probabilistic reward task (PRT) experiment in the EMBARC study, Dr. Wang’s team propose a semiparametric inverse reinforcement learning (RL) approach to characterize the reward-based decision-making of MDD patients. The model assumes that a subject’s decision-making process is updated based on a reward prediction error weighted by the subject-specific learning rate. To account for the fact that one favors a decision leading to a potentially high reward, but this decision process is not necessarily linear, they model reward sensitivity with a nondecreasing and nonlinear function. They apply the proposed method to EMBARC study and find that MDD and control groups have similar learning rates but different reward sensitivity functions. There is strong statistical evidence that reward sensitivity functions have nonlinear forms. Using additional brain imaging data in the same study, they find that both reward sensitivity and learning rate are associated with brain activities in the negative affect circuitry under an emotional conflict task.

Guo, X., Zeng, D., & Wang, Y. (2024). A Semiparametric Inverse Reinforcement Learning Approach to Characterize Decision Making for Mental Disorders. Journal of the American Statistical Association, 119(545), 27–38. https://doi.org/10.1080/01621459.2023.2261184